Rethinking the Knowledge Distillation From the Perspective of Model Calibration
Albert Gu, Karan Goel, Christopher Ré, ICLR 2022
Summary
This paper builds up on the idea that more accurate teachers are not better teachers due to a mismatch in abilities. They go on to analyze these ideas through experiments on toy datasets. They confirm this observation and propose a simple calibration technique to address this. Results follow their hypothesis.
Contributions
- They found that the larger teacher model may be too over-confident, so the student model cannot effectively imitate it.
- While, after simple model calibration of the teacher model, the size of the teacher model has a positive correlation with the performance of the student model
Method
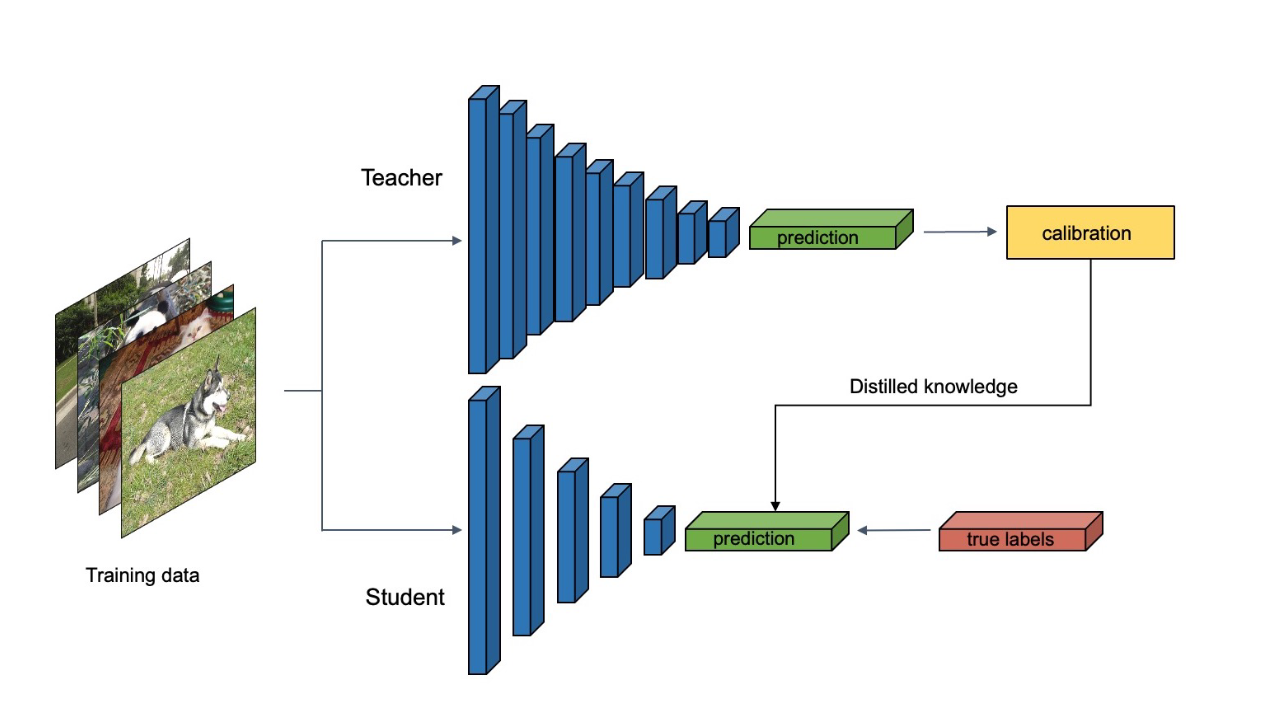
- To analyze the effects of Model calibration, they take student and teacher as CNN models
Resnet.
- To address the over-confident issue, they minimize the negative log-likelihood (NLL) to train the temperature parameter $T$ , and use the temperature scale method to perform simple post-processing calibration on the model
\(\begin{aligned}
\mathcal{L} &=-\sum_{i=1}^{n} \log \left(\hat{\pi}\left(y_{i} \mid \mathbf{x}_{i}\right)\right) \\
\hat{q}_{i} &=\max _{k} \sigma_{\mathrm{SM}}\left(\mathbf{z}_{i} / T\right)^{(k)}
\end{aligned}\)
- $q_i$ is the calibrated confidance
- They are simply changing the original knowledge temprature $t$ to some optimal learned temprature $T$
Results
- Done on
CIFAR-10
and Fashion-MNIST
- They use ECE
Expected Calibration Error
as the matrix. ECE values can be used to calibrate (adjust) a neural network model so that output pseudo-probabilities more closely match the actual probabilities of a correct prediction
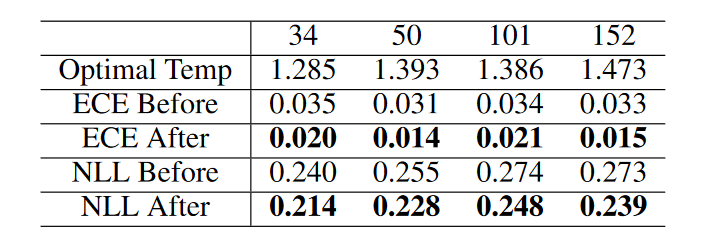
- Results confirm that ECE and NLL go down after calibration. These are also reflected in the accuracy of the student model
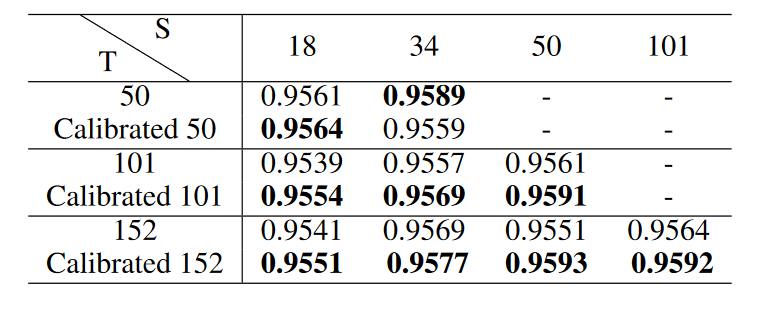
Two Cents
- This paper offers confirms the observation that a bigger student model doesn’t directly imply better student training
- Simply changing the original knowledge temperature to a learned temperature shows improvement
- It’s observed that the model depth width, weight decay, and batch normalization are also essential factors in model calibration, this paper doesn’t take this into account. There’s scope here
Resources